Explore the thrilling journey of AI from the chilling depths of AI Winter to Resurgence. Learn about the setbacks that once threatened progress and the groundbreaking breakthroughs that have reignited hope and innovation.
Introduction
The journey of artificial intelligence (AI) has been exciting. It started with high hopes, hit a low during AI Winters, and now is experiencing a resurgence. The story of AI is filled with ups and downs.
This blog post will take you through AI’s journey. We’ll look at the setbacks and the breakthroughs that have brought back hope and innovation.
You want a clear and engaging story of AI’s journey. You want to learn from past mistakes and understand the resurgence. You also want content that is both informative and relatable.
This blog post will provide you with:
- A detailed explanation of AI Winter and its historical context.
- An analysis of the key setbacks and challenges faced during AI Winter.
- Insights into the factors that contributed to the resurgence of AI.
- Examples of significant breakthroughs and their impact on various industries.
- Lessons learned from past experiences and predictions for the future of AI.
By the end of this post, you’ll understand AI’s journey better. You’ll be excited for what the future holds. Let’s explore AI’s journey together, from AI Winter to AI Resurgence.
Understanding AI Winter to Resurgence
The term AI Winter might sound chilling. Indeed, it was a cold period for artificial intelligence. This section aims to explain what AI Winter was, why it happened, and its impact on AI.
Definition and Historical Context
AI Winter refers to periods when interest and funding in AI research declined. These winters occurred in the 1970s-1980s and the early 2000s.
During these times, the initial excitement for AI was met with disappointing results. This led to a loss of confidence and support.
Early Optimism and Subsequent Disillusionment
In the early days, AI was seen as revolutionary. Researchers and the public believed AI would soon achieve remarkable feats.
However, as the technology’s limitations became clear, hopes turned to disillusionment. The AI systems of the time struggled with basic tasks, widening the gap between expectations and reality.
Key Periods of AI Winter
- 1970s-1980s: The first major AI Winter occurred when the initial hype around AI could not be sustained. Projects like the General Problem Solver and early neural networks failed to deliver on their promises. Funding agencies and governments, disappointed by the lack of progress, cut back on their support.
- Early 2000s: A second AI Winter hit when the limitations of expert systems and symbolic AI became evident. Despite some successes, these systems were brittle and could not handle the complexity of real-world problems. This led to another round of funding cuts and a decline in research activity.
Over-Promising and Under-Delivering
One reason for AI Winter was over-promising and under-delivering. Researchers and companies made bold claims about AI’s capabilities, which were not backed by the technology of the time. When these promises were not met, it led to disappointment and a loss of trust.
Technical Limitations and Funding Cuts
The technology of the time was not ready for AI’s ambitious goals. Computing power was limited, algorithms were not advanced enough, and there was a lack of data to train AI systems effectively.
These technical limitations, combined with high expectations, led to a cycle of hype and disappointment. As a result, funding agencies and investors pulled back, slowing down research and development.
Key Setbacks During AI Winter
The AI Winter was filled with many challenges. These obstacles tested the determination of those working in AI. Looking back, we see how hard it was to keep going.
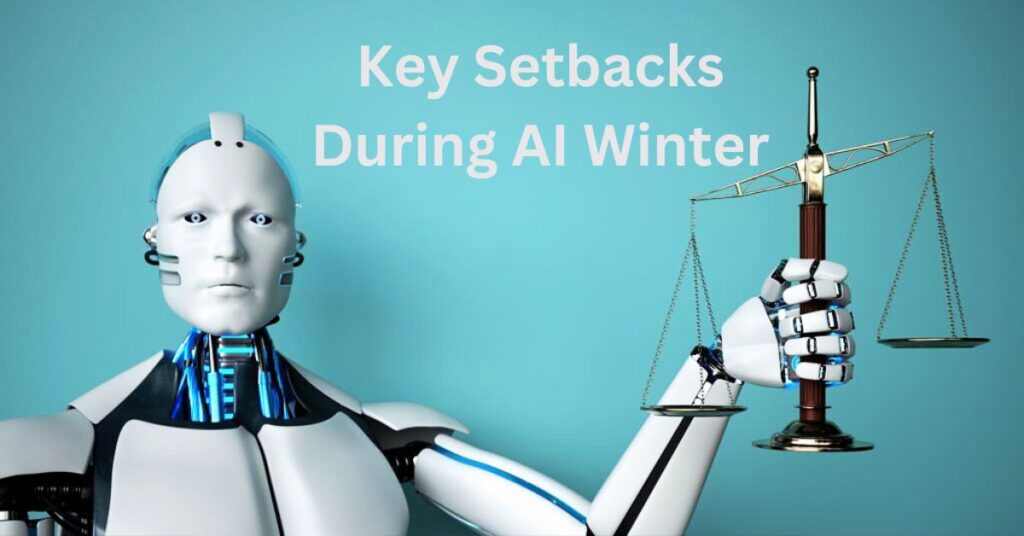
We know that the technical and historical aspects of AI can be complex. You might be struggling to see how these setbacks impacted the development of AI and why they were so significant.
You need a clear, relatable explanation of the key setbacks during AI Winter. You want to understand the human side of these challenges and how they influenced the direction of AI research.
Technological and Research Challenges
AI Winter brought big challenges in tech and research. The computers and algorithms back then were not up to the task. Early AI systems found it hard to do simple tasks, and the gap between hope and reality grew.
- Limitations in Computing Power and Algorithms: The computers were not strong enough for AI’s complex needs. The algorithms were still young and not ready for real-world problems.
- Notable Projects that Failed to Meet Expectations: Big AI projects, like the General Problem Solver and early neural networks, didn’t live up to their promises. These failures made people lose faith and support.
Over-Promising and Under-Delivering
AI Winter was also caused by too much hype and not enough delivery. People made big claims about AI that it couldn’t live up to. When these promises were broken, trust was lost.
Case Studies of Failed AI Projects: The Lighthill Report in the UK and the ALPAC report in the US showed AI’s weaknesses. They led to big cuts in funding. These reports said AI wasn’t practical and was moving too slowly.
Impact on Public Perception and Funding: The repeated failures made people lose interest in AI. Funding agencies and investors backed off, slowing down research and development.
Technical Limitations and Funding Cuts
The tech of the time couldn’t meet AI’s high goals. Computers were weak, algorithms were basic, and there was little data. These tech limits and high hopes led to a cycle of hype and disappointment.
Impact on Research and Development: Without funding, AI research slowed down a lot. Many experts left, and progress was stuck. This cold period was a big setback for AI, delaying many breakthroughs.
The Resurgence of AI
After AI Winter’s cold and tough times, AI started to thaw and grow again. This comeback was thanks to several important factors that brought back interest and money in AI. Today, we see amazing AI advancements.
Factors Leading to Resurgence
The comeback of AI wasn’t sudden but a slow process. Several key factors helped create a good environment for AI research and development. This led to the big wins we see today.
Advances in Machine Learning and Neural Networks: New machine learning and neural networks were a big help. These allowed AI to learn and get better over time, making it more useful and flexible.
Increased Computational Power and Data Availability: Better computers and more data were key in AI’s comeback. These helped researchers train more complex models and get better results.
Key Breakthroughs: Big wins in deep learning and reinforcement learning were crucial. These techniques let AI systems handle harder tasks and achieve great results in areas like image recognition, natural language processing, and games.
Significant Achievements Post-Resurgence
The comeback of AI brought about many big wins. These showed AI’s power and brought back interest and money into the field.
Development of Generative AI and Its Applications: Generative AI, which makes new stuff like images and music, is a big deal. It’s used in many areas, from art to science.
Success Stories in Various Industries: AI has helped many fields, like health, finance, and transport. These stories show AI’s real value and encourage more work and money.
Role of Big Data and Cloud Computing
Big data and cloud computing helped AI come back strong. They gave AI the power to solve tough problems and get better results.
Impact on Research and Development: Big data and cloud computing sped up AI research. They let scientists try new things and push AI’s limits.
Breakthroughs in Modern AI
AI’s comeback has led to big wins that changed many industries and our lives. These wins show AI’s amazing potential and the progress since AI’s tough times.
Development of Generative AI and Its Applications
Generative AI is a big deal in modern AI. It can make new things like images and music, opening up new possibilities.
Generative AI in Creative Industries: Generative AI has changed creative fields. It lets artists, musicians, and writers try new things. AI art and music are getting popular, showing its creative side.
Scientific Research and Innovation: In science, generative AI helps simulate complex things and come up with new ideas. This has helped me find new things in drug-making and materials science.
Success Stories in Various Industries
AI has worked well in many areas, showing its real benefits. This has brought more money and research into AI.
- Healthcare: AI has changed health by improving diagnosis and treatment plans. AI can look at medical images well, helping doctors find diseases early.
- Finance: In finance, AI helps spot fraud, manage risks, and trade algorithms. These uses have made financial dealings safer and more efficient.
- Transportation: AI has made big steps in transport, especially with self-driving cars. These cars use AI to drive safely and avoid obstacles.
Key Breakthroughs in AI Techniques
Several key AI techniques have helped AI get better at solving hard problems. These techniques have led to impressive results.
- Deep Learning: Deep learning, a part of machine learning, has been key. It uses neural networks to learn from lots of data and make good predictions.
- Reinforcement Learning: Reinforcement learning lets AI learn from trying things and getting better. It’s used for AI to play games, control robots, and optimize systems.
Future Potential and Ongoing Research
The recent AI breakthroughs have opened up new possibilities for the future. Research is exploring new areas.
- AI in Everyday Life: AI is becoming a big part of our daily lives. It’s in virtual assistants and smart home devices, making things easier and more efficient.
- Ethical and Responsible AI: As AI grows, making sure it’s fair and responsible is important. Researchers are working on making AI systems that are transparent and value human ethics.
Lessons Learned and Future Outlook
The journey of AI has seen ups and downs, from the AI Winter to its current rise. These experiences teach us valuable lessons for the future. By looking back, we can better face challenges and keep pushing AI forward.
Insights from Past AI Winters
The AI Winters were times of high hopes followed by big disappointments. We’ve learned to manage our expectations and set achievable goals.
- Managing Expectations: AI Winter taught us the importance of being realistic. Over-promising led to disappointment. Today, we must share what AI can and can’t do.
- Sustainable Funding and Support: The funding drops during AI Winter showed us the need for steady support. Keeping AI research funded is key to progress and innovation.
Importance of Interdisciplinary Collaboration
AI research has grown thanks to teamwork across fields. Insights from computer science, neuroscience, and more have led to big AI advances.
- Collaborative Research: Working together across disciplines leads to better solutions. It helps us tackle complex problems and advance AI research.
Ethical and Responsible AI Development
As AI grows, focusing on ethics is crucial. We must ensure AI is fair, and transparent, and values human ethics to build trust and maximize benefits.
- Fairness and Transparency: Creating AI that’s fair and open helps avoid biases. It ensures AI benefits everyone. Developers must prioritize these values for trustworthy AI.
- Alignment with Human Values: AI should respect human values and ethics. This means considering its social and economic effects. It should positively impact society.
Predictions for the Future of AI
The future of AI looks bright. Ongoing research will bring more breakthroughs and uses.
- AI in Everyday Life: AI will become more part of our daily lives, making things easier and more efficient. It will improve our lives in many ways, from smart homes to healthcare.
- Advancements in AI Techniques: New AI methods, like deep learning, will help AI solve harder tasks. This will open new doors in robotics, language processing, and more.
- Focus on Ethical AI: Ethical AI development will get more attention. There will be more talks on how to use AI responsibly. This will involve discussions between researchers, policymakers, and the public.
By learning from the past and focusing on ethics, AI can continue to grow for the good of society. Let’s move forward with a deep understanding of history, ready to embrace AI’s future with optimism and a commitment to positive change.
Conclusion
The journey of artificial intelligence is a story of human resilience and innovation. We’ve seen the highs and lows, the setbacks and breakthroughs. It’s a tale of people who believed in AI, even when it seemed impossible.
Looking back, we learn the value of managing expectations and setting realistic goals. AI Winter taught us to be careful with our promises. We must build trust through honesty and transparency.
Progress in AI comes from working together. It needs diverse perspectives and consistent support. Sustainable funding and collaboration are key to moving forward.
AI’s future is full of possibilities. It can change healthcare, finance, transportation, and more. But we must use AI responsibly and ethically.
Let’s move forward with a deep understanding of AI’s past. We should focus on ethical development. This way, AI can make our future brighter and more inclusive.
Thank you for joining us on this journey. Stay curious and informed. Let’s explore the amazing possibilities ahead.